Tough Quality Problems in Manufacturing
- Chris Butterworth
- Apr 14, 2024
- 5 min read
Manufacturing companies deal with quality problems routinely. The simple ones are easily corrected and so they tend not to draw a lot of attention. But there are also complex problems that do attract attention as they generate defects every day. Unfortunately, many of these go uncorrected for months and even years. These are the sort of problems that eat up much, often most, of the profits of the company. When teams tackle these problems and are unsuccessful after several attempts, they give up. It is assumed that the problem is just too complex.
In my experience, it is very rare that a root cause of a quality problem is a second-order relationship with an upstream variable or an unexpected interaction between two variables. It is, for the most part, simply a variable that isn't currently on your list or in your head. When the problem is eventually solved, it will turn out to be something rather simple and, in hindsight, somewhat obvious. You just haven't reached it yet. It's just around the corner and you will discover it, so stay the course.
Why is it that some problems prove to be very difficult to detect? What makes them so special? Let's take a really close look at what is going on inside the production processes.
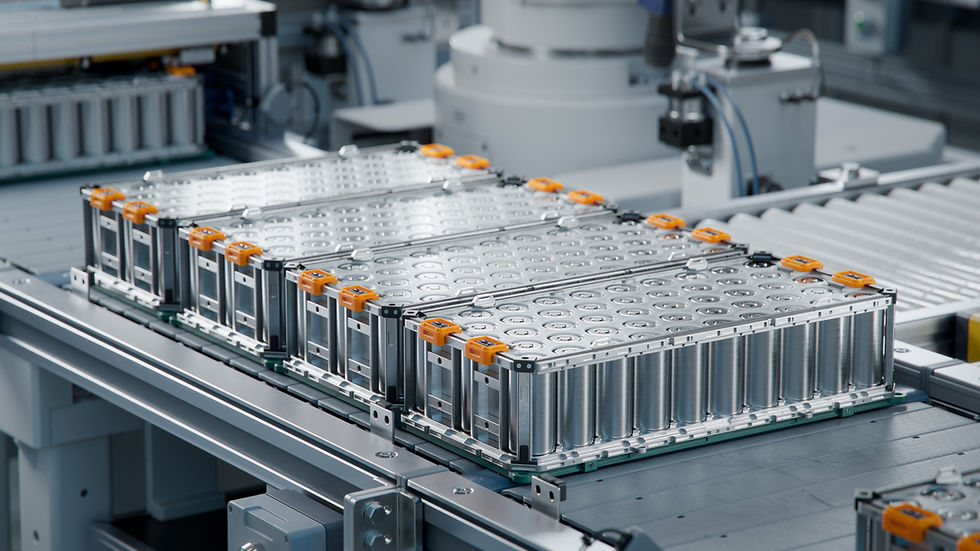
Manufacturing processes either add material (e.g., assembly, welding, blending, mixing, etc), remove material (e.g., machining, etching, cutting, punching, etc ) or deform material (e.g., injection moulding, stamping, extruding, roll-forming, etc). This is accomplished with parts and materials moving through a process, guided with holders and presented to machinery for processing.
Consider all the various production equipment in your factory. All the hardware items such as fixtures, holders, guides, feeders, rollers, dies, moulds and more. There are no perfect geometries. Every item is a little different than what the design called for. The only place that your press roller is perfectly cylindrical is on the drawing that the design engineer developed. The actual roller is cut from steel stock and that's a rough process. Even after machining and grinding, the press roller in your factory is not perfectly round, the two ends sit at slightly different heights, it isn't perfectly concentric and a close inspection of the surface reveals other imperfections. And that's just one item. They're all like that. In many factories, the products are carried in holders as they are presented to equipment for processing. These holders all vary by some amount. So the products, as they are presented to the equipment, all sit at slightly different positions and through that, receive slightly different processing. That's the effect of variability amongst all your hardware items.
Now consider all the process parameters. Every pressure, flow rate, conveyor speed, oven temperature, current and more, all vary. These things vary in the short term - what is typically considered noise or unexplained variation. They also vary in the medium and long term. These patterns of variation often show cyclic patterns, trending and shifting as well as occasional spikes and dips.
Add to all this the fact that, over time, everything is wearing out.
Now, try to imagine this complex geometry of many sources of variation acting on your product. Some of them change gradually while others change abruptly. Some changes are periodic. You can observe them when isolated and they may display a repeating pattern. Others are immediate changes. Imagine that every 12th holder is thinner by 0.001". Every 12th part is now being processed differently.
There are also variables that require some thorough digging in order to reveal their patterns. Consider a worn hardware item that is prone to slipping. A brand new item never slips but a worn item can slip, or it might not slip. When it does slip, it does so by a different amount every time. Worn items are difficult to pinpoint for this reason.
This is a cacophony of multiple sources of variation all changing with their own patterns. It's no surprise that problem solving in manufacturing can be difficult. There are many variables to consider.
In many cases, the extent of variation is small enough to be tolerated. We build and ship products under these conditions all the time. But when you start to see an increase in a defect, or a new defect type altogether, consider all the sources of variation affecting that part. List them out and go and study them.
We can learn a lot about process behaviour by plotting the data that the process generates. Graphs are our window into the world of industrial processes. As you start to collect data and review the charts generated, you will observe unexpected patterns. These can be clues and they can help you on your way to a root cause. Here's an example;
The company had a quality problem which they were unable to solve, despite several attempts. The problem was a failure at final testing where a performance curve did not resemble the ideal curve that was the test standard. The team was unable to diagnose the reason for the slight dip in the curve, so they decided to reject those products and keep an eye on things. As a result, every batch had 2 to 5 % failures.
Unable to solve the problem using the data sets available, we went into an upstream process and captured data from a different test. Here, we selected particular locations where measurements were taken and added them all to a new file. Included in that file were the measured data points, differences between measurements, range of measurements, times between measurements etc. It was one particular plot where a pattern emerged that was worth investigating.
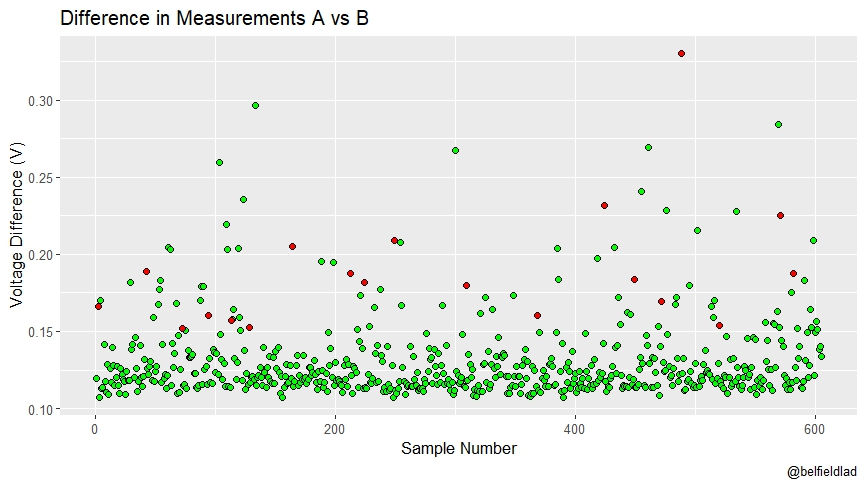
The difference between two measurements was mostly under 150 mV and there were a few outliers in the data (green = good, red = bad) . The interesting feature here is that, although good and bad parts were in the tail area (beyond 150 mV), bad parts were always in the tail area and never in the main part of the distribution (under 150 mV).
This was a critical piece of insight and provided a clue, not yet a solution. Why would failures exhibit this feature? Later, when we looked closely at the test equipment, we were able to connect the dots to the chart above. A worn component that had not been replaced since the equipment was new, was responsible for the difference in measurements. This difference manifested in a nonconforming performance curve at final testing.
Connecting the problem to the source is satisfying work and in this particular problem, it was exciting. The source of the problem had eluded detection for a long time. This was several years ago and the defect has not returned.
Problem solving is detective work and your primary sources of information are plots of data. When a problem seems really tough and you've exhausted all your searches, keep going. There may be additional data hidden in some other files. Look at upstream data including process data as well as product measurements. Timestamps can provide insight that the brainstorming sessions don't generate. You should also measure additional features of the product that you haven't yet measured. A correlated variable can also lead you to a root cause.
There's a lot about problem solving that you need to know to be successful.
Σχόλια